In the ever-evolving landscape of artificial intelligence, the quest for achieving more sophisticated and precise responses from generative AI models has been an ongoing pursuit. The latest milestone in this journey comes from Google DeepMind researchers and Princeton University, who have introduced an innovative prompting strategy known as Tree of Thoughts (ToT). This groundbreaking approach surpasses traditional methods and unlocks new dimensions of reasoning within language models, offering a promising avenue for tackling complex problems that require search or planning.
Understanding the Significance of Tree of Thoughts (ToT)
The Evolution from Chain of Thoughts (CoT)
Before delving into the intricacies of ToT, it’s essential to grasp its evolution from the popular Chain of Thoughts (CoT) method. CoT focuses on guiding a language model through a logical sequence of thoughts to generate coherent and connected responses. It relies on a linear path, resembling the fast and automatic decision-making process known as “System 1” in human cognition.
In contrast, ToT adopts a more deliberative approach, akin to the “System 2” cognitive model, urging the language model to follow a series of steps while introducing an evaluator step at each stage. This evaluator critically assesses the viability of each step and determines whether it contributes to reaching a final solution. If a particular reasoning path proves inefficient, ToT allows the model to abandon it and explore alternative branches, mirroring the kind of heuristic-guided search seen in human problem-solving.
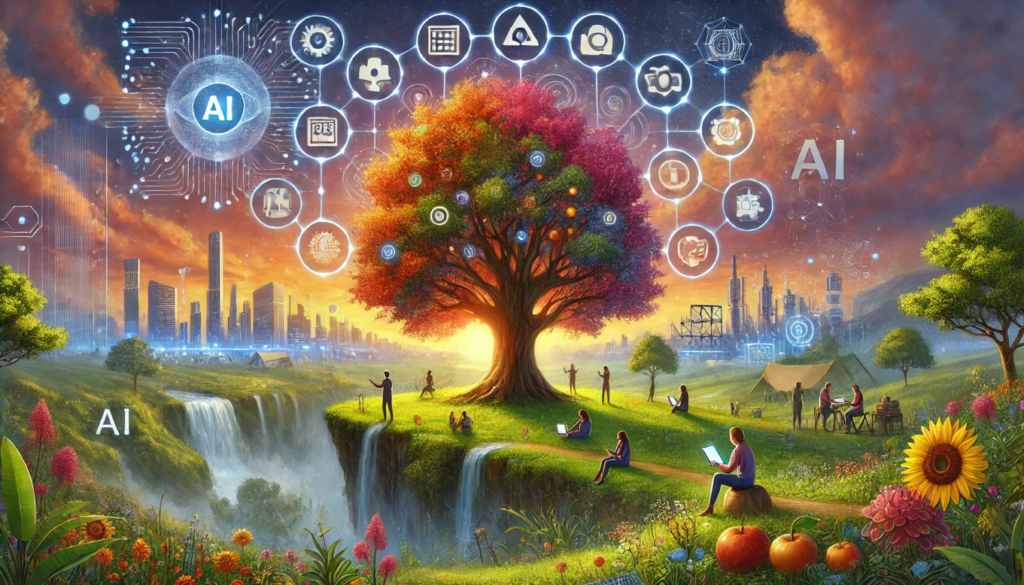
Dual Process Models in Human Cognition
ToT draws inspiration from the dual process models in human cognition, which propose that humans engage in two distinct decision-making processes:
Fast, Automatic, Unconscious: This mode relies on intuition, resulting in fast and automatic decision-making.Slow, Deliberate, Conscious: In contrast, this mode involves careful consideration, analysis, and step-by-step reasoning before arriving at a final decision.
ToT’s unique feature is its utilization of a tree structure to represent each step of the reasoning process. This allows the language model to evaluate each reasoning step, mimicking the deliberative “System 2” thinking. If a step is deemed unproductive, ToT prompts the model to explore alternative paths until a satisfactory answer is achieved.
Comparing ToT Against Other Prompting Strategies
To gain a comprehensive understanding of ToT’s effectiveness, it’s crucial to compare it against other prominent prompting strategies. The research paper presents a comparative analysis of ToT with three key approaches:
Input-Output (IO) Prompting: This method involves providing the language model with a problem to solve and obtaining the answer. It’s commonly used for tasks such as text summarization.
- Example:
- Input Prompt: Summarize the following article.
- Output Prompt: Summary based on the input article.Chain of Thought Prompting (CoT): CoT guides the language model through a logical sequence of thoughts, enabling it to solve problems by following intermediate reasoning steps.
- Example:
- Question: Roger has 5 tennis balls. He buys 2 more cans of tennis balls. Each can has 3 tennis balls. How many tennis balls does he have now?
- Reasoning: Roger started with 5 balls. 2 cans of 3 tennis balls each is 6 tennis balls. 5 + 6 = 11. The answer: 11Self-Consistency with CoT: This strategy prompts the language model multiple times and selects the most commonly arrived at answer. It acknowledges the existence of multiple valid reasoning paths.
- Example:
- Example:
ToT’s superiority lies in its ability to explore various reasoning paths, both locally and globally, while incorporating planning, lookahead, and backtracking—a distinctive feature that aligns with human problem-solving.
Real-Life Application: Testing ToT with a Mathematical Game
To validate the effectiveness of ToT, the researchers conducted tests using a mathematical card game known as the Game of 24. In this game, players must use four numbers from a set of cards, each usable only once, and combine them using basic arithmetic operations to achieve a result of 24. The results of these tests consistently favored ToT over the other prompting strategies.
Conclusion: A New Horizon in AI Prompting
In conclusion, Tree of Thoughts (ToT) has emerged as a transformative breakthrough in the realm of AI prompting. It bridges the gap between the intuitive, fast decision-making processes of “System 1” and the deliberate, conscious thinking of “System 2.” By introducing a tree and branch framework, ToT empowers language models to explore multiple reasoning paths, ultimately leading to more sophisticated and promising solutions.
While ToT presents remarkable potential for enhancing AI capabilities, it’s important to note that it may not be necessary for tasks that GPT-4 and similar models already excel at. Nevertheless, the intersection of language models with classical approaches to AI marks an exciting direction, offering new avenues for solving complex problems, including creative writing.
For those interested in delving deeper into the details of Tree of Thoughts, the original research paper, titled “Tree of Thoughts: Deliberate Problem Solving with Large Language Models,” provides a comprehensive resource.